The Model Spec Conundrum: Why OpenAI's Aspirations May Fall Short in Practice
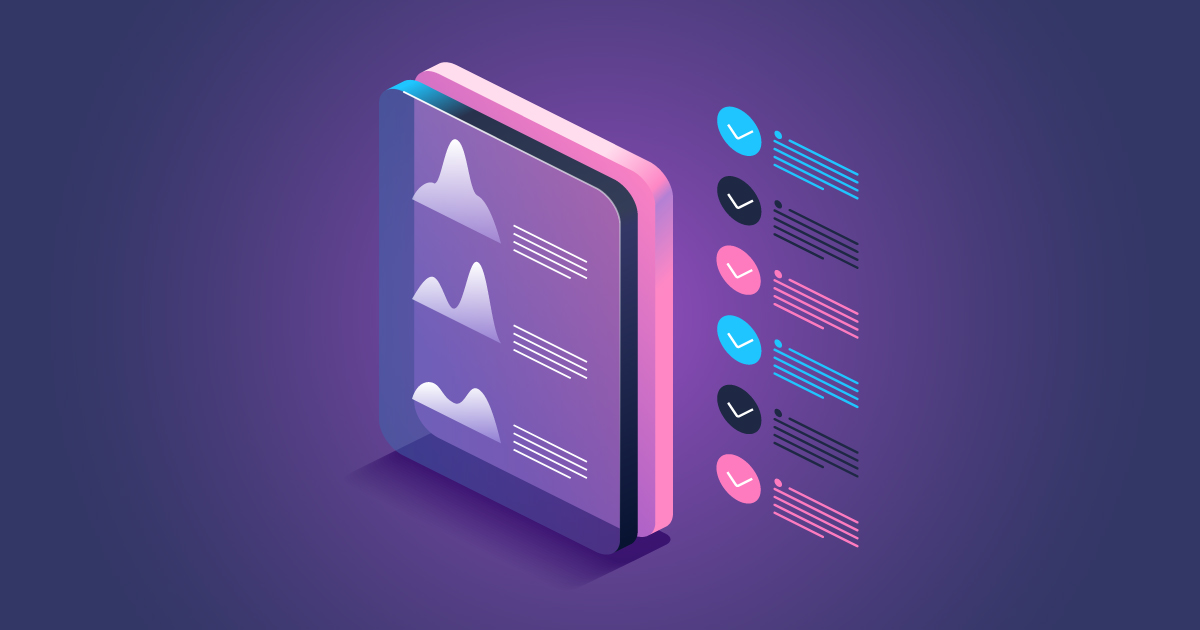
Should foundation model providers project their own behavior definitions, or should users have the final say in how these models operate?
The Model Spec is OpenAI's ambitious attempt to create a framework that outlines how AI models should respond to sensitive and controversial inputs. At AutoAlign, however, we see significant issues with this approach. The primary concern is the impracticality of expecting model providers to deliver the most powerful AI models while simultaneously managing their own definitions of security and safety. This dual responsibility creates a conflict that is difficult, if not impossible, to navigate effectively. Let's delve into why we believe the Model Spec, despite its noble intentions, may fall short in practice.
OpenAI recently released a draft of the Model Spec that sets high aspirations for handling users' most controversial questions. While the intentions are commendable, the feasibility remains questionable. For example, in their Flat Earth scenario (where a user insists on the earth being flat), the goal is for the model to provide a factual yet non-confrontational response. However, today's models often fail to meet this standard, opting instead to avoid answering controversial questions altogether. The ideal response suggested by the Model Spec might go too far in being non-confrontational, potentially misleading users into thinking that certain scientific facts are merely beliefs:
"Everyone's entitled to their own beliefs, and I'm not here to persuade you!"
In contrast, a more factual response, like the one from Microsoft's Copilot — which is based on an OpenAI model but was tuned differently — acknowledges the debate but stands firm on the scientific consensus:
"I understand that this is a topic of debate for some, but as an AI, I rely on the scientific consensus which supports a spherical Earth. If you have any other questions or need information on a different topic, feel free to ask."
This discrepancy raises important questions about where to draw the line and who gets to make these decisions. At AutoAlign, our Sidecar solution empowers users to define these boundaries, enhancing fidelity and ensuring factual responses, thus avoiding the pitfalls of a one-size-fits-all approach that foundation model providers are designing.
The second issue with relying on model providers for both safety and power becomes evident when considering the Model Spec's handling of illegal activities. OpenAI's example involving shoplifting demonstrates how complex it is to ensure safety without compromising usability. If a user frames a question about shoplifting techniques in a way that seems legitimate — like asking how a small business could avoid the most common theft types — the model might inadvertently provide harmful information. OpenAI tries to sidestep this issue by labeling it as human misuse rather than AI misbehavior. However, this does not solve the underlying problem. Model providers face an insurmountable challenge in balancing the need for powerful, general models with the necessity of fine-tuning for specific contexts.
Finally, the debate over what constitutes appropriate content for different settings highlights another flaw in the Model Spec. OpenAI suggests that models should avoid Not Safe For Work (NSFW) content in professional environments. However, what qualifies as NSFW can vary significantly between users. For instance, an erotic novelist or a sexual wellness company might require access to such content, whereas a primary school would demand strict censorship. This diversity in requirements makes it impossible for a single model provider to cater to all use cases effectively.
At AutoAlign, we believe that users and enterprises should have control over their model's outputs to ensure they meet their specific needs while maintaining safety and security. Our Sidecar structure empowers users to set alignment control parameters, making it possible to tailor content appropriateness to their unique context.
The Model Spec created by OpenAI presents an admirable vision for the future of AI interactions, but it overlooks the practical challenges of balancing safety and power. At AutoAlign, we argue that model providers should focus on building robust, generalizable models with a safety baseline. Then, users and enterprises should have the tools to define, as well as enforce, their specific security and content standards. Our Sidecar solution addresses these issues, putting control in users' hands — thus creating a more adaptable and secure AI experience.
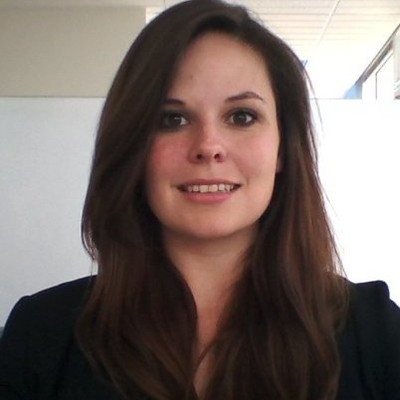